Data Sourcing: The Key to Any Alternative Data Initiative
Background
As asset managers invest resources into building out alternative data initiatives, data sourcing is seen as an integral part of the process, with in-house and outsourced professionals finding and introducing datasets and data vendors. With datasets rapidly growing in price (figure 1) and impacting the growth in spending of firms buying alternative data (figure 2), the data sourcing function is now more critical than ever before. These results are based on our first-of-its-kind survey of 24 buyside funds with varying levels of alternative data experience.
We are currently running our 2022 alternative data survey for buyside funds following the success of last year’s initiative – to learn more about this year’s survey report and submit your response click here.
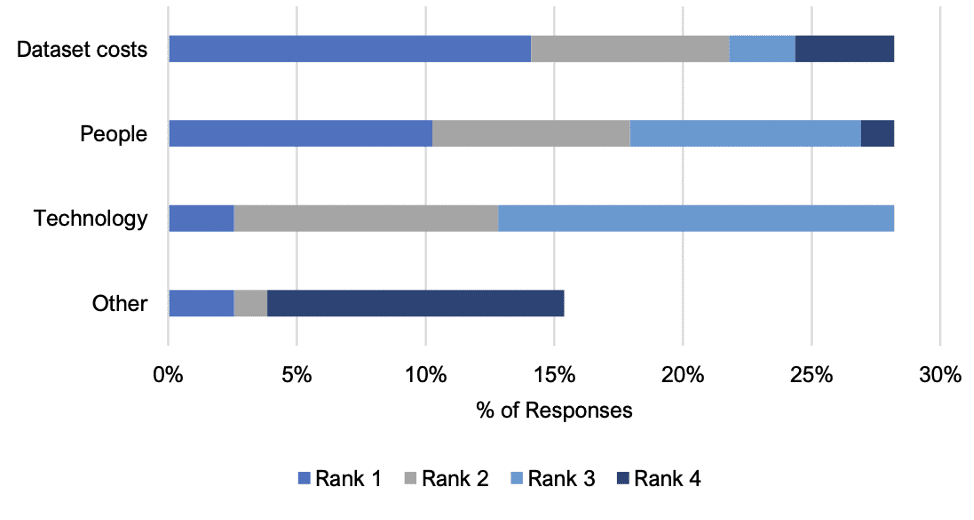
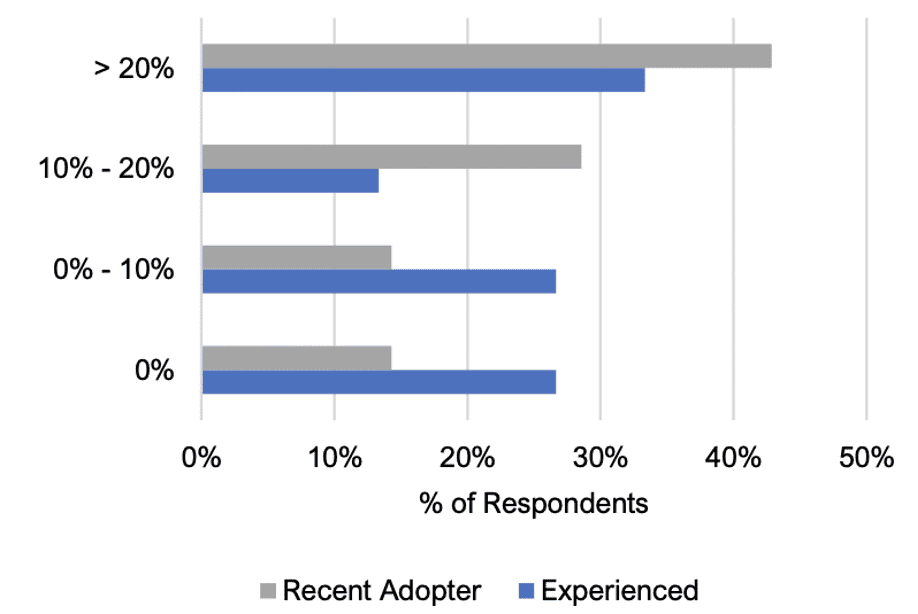
Experienced firms are more likely to have dedicated employees who are focusing on data sourcing and data procurement. Interestingly, average dataset costs were lower for experienced funds compared to recent adopters. The reason behind a higher cost for recent adopters could be due to a lack of experience negotiating dataset prices or a focus on more expensive ‘core’ datasets rather than on adding lower-priced incremental datasets.
The importance of a data sourcing function within data teams was confirmed by The Economist’s Intelligence Unit, which conducted a broad survey of 914 executives from eight industries and across 13 countries. The Economist’s 2021 survey respondents stated that budgetary increases were expected over the next three years in data analytics (85%), data storage (83%), and buying data from third-party data vendors (72%).
According to Nasdaq, outsourcing of the data procurement function was also highlighted in the survey (more details below) and alternative data aggregators with their advisory groups serve as an important resource for both experienced firms and early adopters.
A Note on Team and Workflow
For asset managers, market saturation is one of the greatest challenges facing data sourcing teams as finding new and unique datasets will only get tougher. There are six key steps in the data sourcing and management lifecycle.
- Initiation requires defining the motivation and purpose behind the initiative and outlining the roles and responsibilities of the data sourcing team. This includes achieving management buy-in is also a crucial step in the initiation phase.
- Screening and the identification of relevant data sources through avenues like data marketplaces, conferences, and matching different data types with use cases.
- Assessing the data further for provenance and licensing issues, and checking for data quality and consistency issues.
- Following screening and initial assessments, the sourced data needs to be onboarded and mapped in internal systems with access rights clarified. Proper integration of various sources is essential as buyside firms would often try to combine different datasets to find new alpha signals.
- Managing and using the data encompasses ongoing data management monitoring and data quality assurance. Firms also develop data certification processes with transaction logs and audit trails.
- Finally, firms might choose to archive the sourced data for potential repeat use when it is no longer considered valuable or to remove it completely. The increased competition in the alternative data space means that firms constantly research new data sources for cost efficiency and to find a new source of alpha.
In McKinsey’s 2021 report titled “Harnessing the Power of External Data,” they stated that hiring a dedicated data scout/strategist is essential when building an in-house team for external data sourcing. Data scouts and strategists partner with data analytics and engineering departments to focus on specific use cases, identify and prioritize datasets for further investigation, and measure return on investment (ROI). Ideal candidates would have experience in integrating data analytics capabilities and working with both the technology and business functions of a firm.
In BNY Mellon’s report exploring “How Data and Analytics Can Help Investment Managers Future Proof Their Business,” data democratization and generating internal awareness are seen as necessary steps in implementing successful data and analytics strategies. Furthermore, organizations realize that a single solution can’t serve all their data needs: “Many of our clients pursuing transformation initiatives today will cite “vendor lock-in” as one of the prevailing risks they want to avoid.”
With firms adopting data-driven processes at such a high rate, it is no surprise that outsourcing parts of the alternative data process has also become increasingly popular. In our 2021 report exploring the “First Steps in Alternative Data for the Buyside”, firms’ most common mistakes when implementing a new alternative data strategy are attempting to do it all in-house and over-hiring.
In Nasdaq’s survey of 200 asset managers (figure 3), 61% of those satisfied with their firm’s data strategy currently outsource some functions, while 64% of dissatisfied respondents do not outsource at all. Furthermore, several cohorts plan to ramp up outsourcing: 1) firms taking more than a month to onboard and integrate new datasets (71%); 2) PMs under the age of 40 (70%); 3) Organizations who are led by Chief Data Officers (61%).
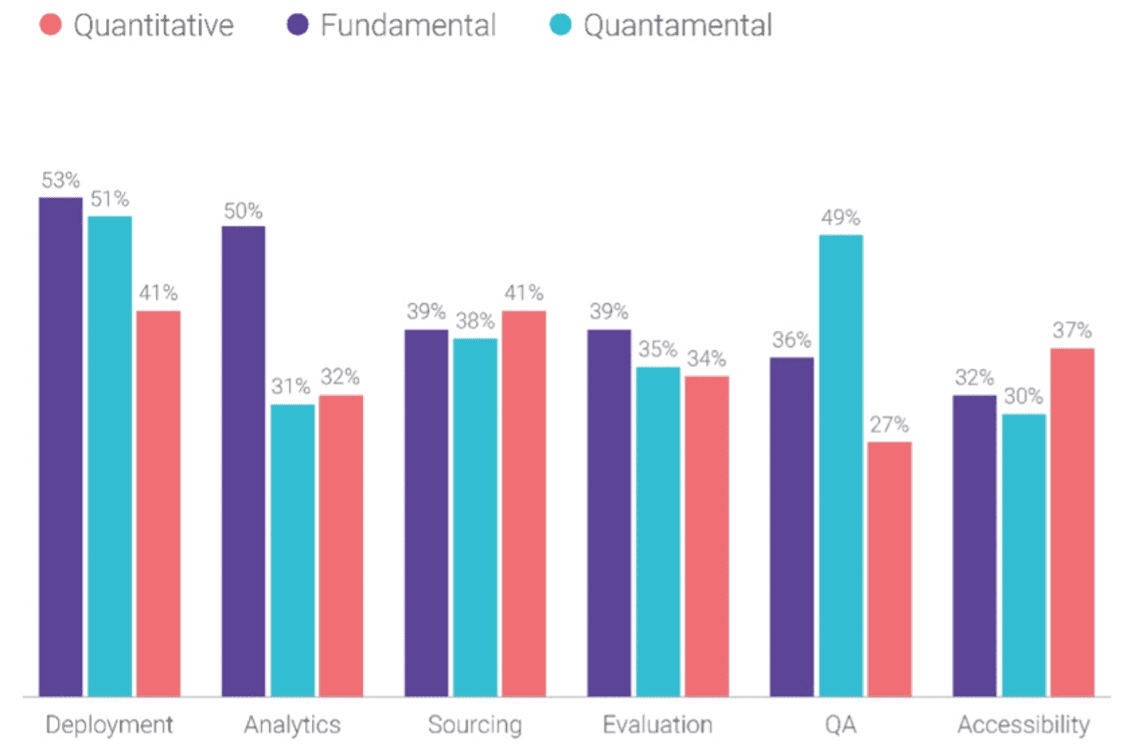
According to McKinsey, data sourcing teams are advised to develop relationships with data marketplaces and aggregators instead of spending months on vendor discussions and negotiations. Working with data marketplaces “can give organizations ready access to the broader data ecosystem through an intuitive search-oriented platform, allowing organizations to rapidly test dozens or even hundreds of data sets under the auspices of a single contract and negotiation.”
These Are The Challenges, Here Are The Solutions
According to Brightdata, data sourcing challenges need to be evaluated in a more granular way as they are interlinked with data analysis issues:
- Data identification requires spotting and organizing datasets by use cases and asset classes.
- Process replicability means determining whether it is a one-off dataset sourcing or a reliable and consistent data delivery.
- Information quality looks at how clean and traceable a dataset is.
- Since disparate sources can come in various data formats (texts, videos, voice files, images, etc.), this makes it hard to aggregate and cross-reference a data catalog.
Once a potential dataset is identified, McKinsey recommends data engineers and data scientists work with other business stakeholders to evaluate the value added by assessing the following factors:
- Depth and breadth of data: This can include ticker coverage and a variety of use cases available to implement the dataset on.
- Data delivery: There are different ways of transferring data, including API, FTP, Platform, or plain CSV.
- Match/fill rates: This relates to how well a specific metric is covered.
- Potential impact/lift: Firms need to properly backtest and evaluate the dataset ROI.
- Data profile: This can include the analysis of missing variables and the dataset distribution.
- Total cost: Firms need to estimate costs during trials and production stages.
- Coverage/panel overview: This can include the assessment of geography or any biases present in the data.
- Procurement and contracting: Firms need to understand how long it takes to onboard the data.
- Timeliness of data: This relates to the reliability of historical coverage and the update frequency.
- Risk: Firms need to evaluate all legal and compliance considerations before making the purchase decision.
As alternative data becomes more widely used, buyside firms should consider testing certain research questions before making large investments. Of course, vendors would still prefer to sell their full datasets to large buyers rather than high-volume, low-price points. However, the market evolves over time.
Initial profiling, procurement of sample data, ticker mapping, contracting, legal and compliance considerations – data marketplaces and intermediaries like Eagle Alpha can take all these functions on while also helping with identifying and prioritizing data products.
For more intelligence on alternative data teams across the asset management space and to benchmark against your own initiative, our 2022 alternative data survey for buyside funds is now open – to learn more and participate click here.
Final Thoughts
The industry has grown a lot over the last ten years. While credit card data providers priced themselves very high before, with the vendors knowing how valuable the data was, now more players are in the market. Additionally, these alternative data vendors now offer datasets that are already enriched with the necessary metadata. Data quality and ongoing support have also improved; for example, the vendor is now responsible for spotting gaps or breaks in the data whereas the buyer was responsible for this in the past. If you are interested in learning more about our data platform for both data buyers and vendors, please get in touch with our team at inquiries@eaglealpha.com.